The key to success in 2025 will be finding the sweet spot between aggressive AI adoption and sustainable engineering practices.
There’s still a lot of talk in manufacturing circles about artificial intelligence being a flash in the pan technology that’s more sizzle than steak. While that may have been the case back in 2022, things have changed.
AI is not going to replace your workers and make strategic executive decisions. But any manufacturer not carefully considering a well-thought-out AI strategy is leaving money on the table. And it doesn’t matter if you are an enterprise-scale manufacturer or regional company with less than 150 employees, there are plenty of ways to extract value and gain an edge from AI.
“One area where manufacturers can quickly find ROI from AI is real-time knowledge sharing and translation, where AI breaks down language barriers by instantly translating and summarizing documentation like standard operating procedures, repair logs, and shop floor communications,” says Qaiser Habib, Head of Canada Engineering at Snowflake, a cloud data platform developer based out of Bozeman, Montana.
Snowflake has developed a flexible cloud-based platform for modern data teams looking to store, process, and analyze data in a highly scalable and cost-effective manner while enabling collaboration across different departments and organizations. The company has offices in 50 cities around the world. Habib speaks to us from Snowflake’s Canadian engineering hub in Toronto.
“This enables global manufacturing teams [and supply chain partners] to collaborate seamlessly, share best practices across facilities, and access critical operational insights in their native language.”
This is one of several “low-hanging fruit” areas Habib says will drive successful adoption of AI amongst manufacturers.
Manufacturers can also find near-immediate AI ROI is in key areas such as predictive maintenance, where AI monitors equipment to prevent costly unplanned downtime. Additionally, AI guided maintenance solutions can analyze repair logs and equipment manuals to recommend troubleshooting steps faster for more effective repairs.
According to Habib, the key to creating AI ROI is using AI solutions that understand your company and industry-specific processes rather than generic solutions.
AI predictions for manufacturing
Habib made a few predictions about how manufacturers will approach and interact with AI for the next year or so. Here’s a look:
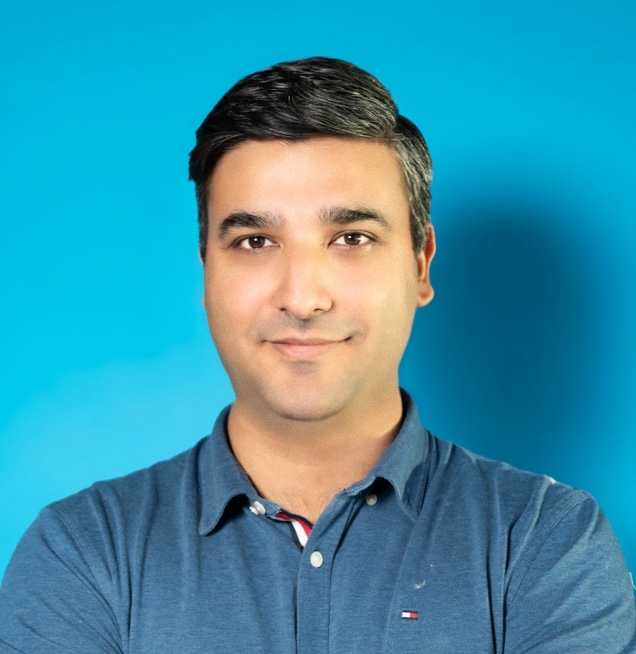
Shift from AI hype to ROI: 2025 will mark the transition from AI experimentation to measurable business impact. 2024 saw widespread hype and experimentation as organizations explored AI and solidified their data strategies. Organizations are now demanding concrete returns, and engineering teams must identify specific, high value problems where AI can deliver measurable results and move beyond proofs of concept to full production systems that emphasize accuracy and reliability. The focus will be on operationalizing large language models (LLMs) and taking evolved approaches to security, governance, and observability.
This shift is particularly evident in the manufacturing industry, where enterprise AI applications are delivering tangible results through computer vision quality control systems. These systems are transforming production by dramatically reducing inspection time compared to manual processes, catching subtle defects that human inspectors might miss, preventing costly downstream quality issues, and enabling 24/7 continuous inspection without fatigue.
Organizations will prioritize AI projects that can demonstrate clear financial impact within specific timeframes, moving away from speculative “AI for AI’s sake” projects toward targeted solutions for well-defined business problems.
Engineers will face the stress test: With attention shifting towards ROI, engineering teams are experiencing unprecedented pressure to validate AI investments while maintaining code quality, security, and team wellbeing. As engineers, we are accustomed to working in fast-paced environments with new technologies and innovations, but there is now added pressure to balance rapid AI implementation with sustainable team practices.
To foster a productive and engaged workforce, leaders must ensure their teams have a crystal clear understanding of the business case behind their AI initiatives and provide the necessary training needed. As organizations prioritize these efforts, upskilling through certification programs and professional development initiatives will remain a critical focus in the workplace.
The key to success in 2025 will be finding the sweet spot between aggressive AI adoption and sustainable engineering practices. Teams that can demonstrate ROI while maintaining team health and code quality will set the standard for the industry, making strategic upskilling and clear business alignment more critical than ever.
AI-as-a-Service will accelerate AI adoption: AI-as-a-Service (AlaaS), which is the delivery of AI tools, apps, and capabilities as a cloud-based service, will emerge as a game-changer in 2025, making AI implementation more accessible and cost-effective for organizations facing adoption hurdles. By removing the need for large upfront investments in hardware and development, AIaaS allows companies to bolt AI capabilities onto existing systems with built-in security and governance features.
Now, instead of requiring developers and engineering teams to build and maintain their own AI systems, they can focus on solving business problems rather than building AI infrastructure from scratch. This also means teams can scale their AI initiatives based on their needs and select AI tools or services tailored to specific use cases. For example, a healthcare provider could use AlaaS to quickly stand up a diagnostic tool that analyzes medical images for early disease detection, while a large retailer can rapidly deploy GenAI-powered chatbots that pull from warranty and return policies to enhance customer service.
The AIaaS revolution also creates new opportunities for engineers to develop and monetize their own AI applications within cloud-based platforms. Similar to how mobile app stores transformed software distribution, AIaaS platforms will enable a new generation of AI-powered startups and create additional revenue streams for developers.
Manufacturing’s AI sweet spot
What does a sweet spot for AI adoption and engineering sustainability look like for a small or medium sized manufacturer that doesn’t have the scale to dedicate an executive to managing this?
“For small and medium manufacturers, the AI adoption sweet spot lies in focusing on practical, high-impact projects with clear ROI and avoiding overly complex, experimental initiatives,” Habib says, citing examples such as using conversational AI interfaces to make ad hoc data and analytics more accessible, creating agents to automate tasks like quality inspections, or implementing retrieval-augmented generation (RAG) systems to search, summarize, and recommend actions from knowledge bases.
At a small manufacturer, RAG systems could be used to improve customer support, technical documentation, or internal knowledge management. For example, an AI-powered chatbot could retrieve relevant technical manuals or past troubleshooting cases to answer customer inquiries more accurately. Additionally, RAG systems could assist with automated reporting or data analysis by generating insights from production data or historical reports, helping the manufacturer make better decisions.
Habib says manufacturers will leverage AIaaS through readily available cloud solutions that integrate with their existing operations. For example, they could use AI-powered demand forecasting services to optimize inventory levels, implement digital twin simulations to test process changes before deployment, or utilize natural language AI to automatically generate technical documentation from production data.
He adds that these solutions can be implemented incrementally, with costs scaling based on actual usage, making AI adoption more manageable and cost-effective than building custom solutions from scratch.