Novel PINN approach achieves high accuracy at reduced computational cost.
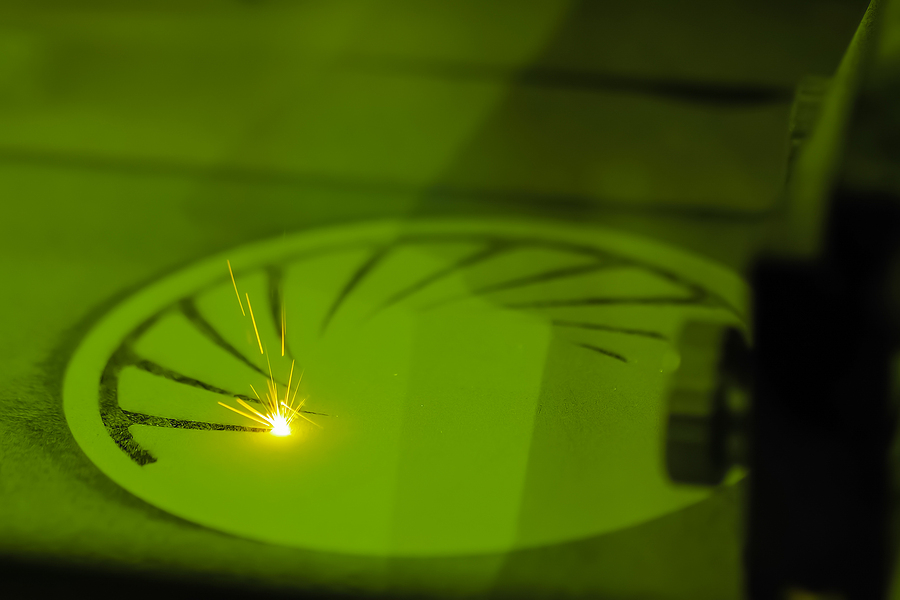
In laser powder bed fusion (L-PBF), also known as direct metal laser sintering (DMLS) or selective laser melting (SLM), the melt pool is where the action is. The high temperature gradients that occur during the process result in non-equilibrium conditions at the solid/liquid interface, which in turn affect the microstructure of the 3D printed part.
The complex physics involved are notoriously difficult to simulate and yet predicting melt pool morphology is critical to ensuring the quality of parts made with metal additive manufacturing (AM). Fortunately, a new approach, pioneered by researchers at Wuhan University, is showing promise in this area. Using a physics-informed neural network (PINN) combined with a machine learning technique known as transfer learning, the researchers have been able to predict melt pool morphology accurately at a significantly lower computation cost compared to conventional simulation.
The so-called transfer learning-enhanced physics-informed neural network (TLE-PINN) combines physical constraints with machine learning, as professor Yaowu Hu, contributing author to the published research, explained in a press release. “By integrating physics-informed modeling with transfer learning, TLE-PINN bridges the gap between traditional numerical simulations and artificial intelligence, offering precise and efficient solutions for predicting melt pool morphology,” he said.
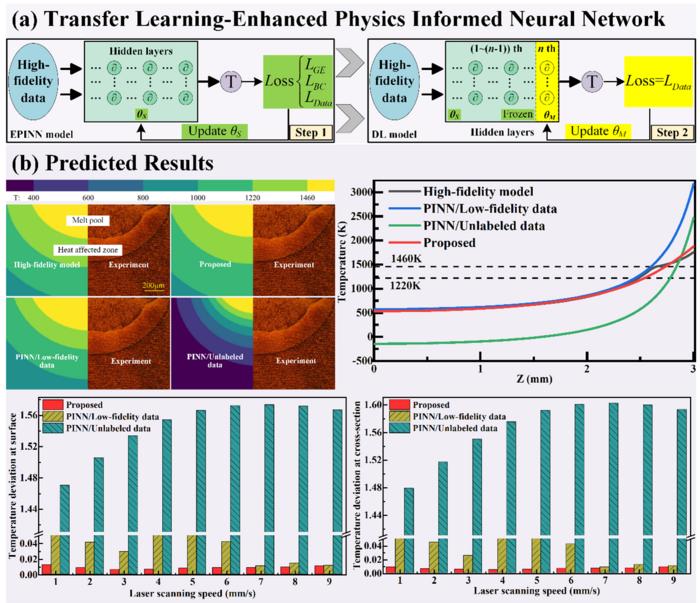
Essentially, the approach incorporates heat transfer equations and boundary conditions into a neural network’s loss function to ensure that it accurately represents melt pool morphology while the transfer learning framework fine tunes the model using high-fidelity data, updating the final layers while freezing earlier network parameters. The result is a neural network that’s more efficiently trained and computationally scalable.
“This design offers a unique combination of speed and accuracy, making it particularly suitable for industrial applications,” said professor Hu.
The researchers validated their framework using 42CrMo steel samples, with the model predicting melt pool morphology across a range of laser scanning speeds: from 1-9mm/s. ompared to traditional PINN and data-driven methods, such as Random Forest and XGBoost, TLE-PINN demonstrated significantly lower temperature deviations and more consistent results.
The researchers believe the efficiency of their approach could enable real-time monitoring of L-PBF in the future and they’re currently exploring ways to expand its capabilities to handle more complex material systems and larger parameter ranges.
The research is published in the journal Advanced Manufacturing.