In the world of digital transformation, data is the most important “currency.”
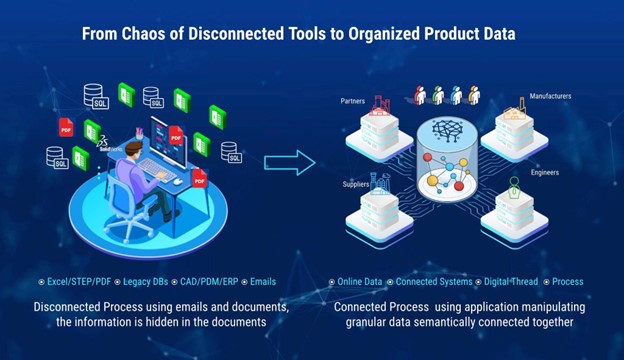
The current software landscape and PLM technologies are limited to providing a mechanism for decision effectiveness and data efficiency. This article explores the critical need for manufacturers to build a comprehensive digital footprint by transforming their approach to data. It examines the challenges posed by traditional methods, the limitations of current solutions and the practical steps required to develop a connected and efficient digital ecosystem. This transformation is vital for achieving smarter processes, operational efficiency and long-term competitiveness in the modern manufacturing landscape.
The complexity of products and business models
Manufacturing is undergoing a significant transformation, driven by the increasing complexity of products and the shift toward digital business models. The changes are not just technical but extend into how companies operate and engage with their customers. At the heart of this evolution is the role of data in enabling more adaptive and efficient processes. From a practical sense, companies are looking at how to build digital interfaces to serve their internal and external processes including communications with suppliers, contractors and customers. All these communications must be “digitized”, which brings many questions about how to do so. What changes are bringing the highest demand for digital transformation?
One of the most significant changes is the rise of Product-as-a-Service (PaaS). In this model, manufacturers move away from selling products outright to offering them as services. For example, a company might provide access to industrial machinery or software on a subscription basis rather than a one-time purchase. This approach not only creates recurring revenue streams but also strengthens customer relationships by allowing manufacturers to stay engaged throughout the product life cycle. However, this model is a nightmare for IT that is required to organize an information stack to support also “service” or “maintenance” data.
Another transformative development is predictive maintenance, which uses data from connected sensors to predict when equipment might fail. This proactive approach reduces downtime, prevents costly repairs and improves operational efficiency. Instead of reacting to issues after they arise, manufacturers can address problems before they impact production. Another great innovation, but it requires a growing amount of data sources (especially from customer sites) to be integrated into holistic data models.
The growing importance of data-driven services has also reshaped the manufacturing businesses from design to production and operations. By analyzing performance data from products in use, manufacturers can offer tailored services such as optimization, upgrades, or customization. This shift not only improves customer satisfaction but also opens new revenue streams.
Outcome-based models represent another innovation, where companies promise specific results rather than just delivering a product. For example, a turbine manufacturer might guarantee a certain level of energy output rather than simply selling the turbine. These models align the manufacturer’s incentives with customer success, creating stronger partnerships.
All these trends point to the growing importance of data in manufacturing. From enabling connected products to providing actionable insights, data lies at the core of modern business models. However, the ability to harness and manage this data effectively remains a significant challenge for many companies.
To support data management transformation, manufacturers are looking at how to adopt new business models and ways to re-tool their enterprise software implementation (including PLM, MES, ERP and others). This requires bringing the need for significant transformation of enterprise data management and modeling that is used by all these tools. How to allow manufacturing companies to access advanced technologies without large upfront investments, especially in existing PLM/ERP software stacks. In other words, how to grow on top of the existing IT and enterprise software layers without replacement of existing tools.
The data problem in manufacturing ecosystems
Despite the growing emphasis on digital transformation, many manufacturing ecosystems remain rooted in outdated practices. A significant portion of manufacturing workflows is still centered around documents – CAD files, PDFs, reports, Excel spreadsheets and information stored on shared drives. While these methods have served the industry for decades, they are increasingly ill-suited to meet the demands of modern manufacturing.
The reliance on documents creates numerous inefficiencies. For instance, files-based content is very difficult to share, search, or update. It is not granular enough and the documents are not easy to link with each other. This leads to duplication of effort, errors in communication and delays in decision-making. Furthermore, document-based systems make it challenging to integrate data across different departments or organizations, resulting in silos of information that hinder collaboration and innovation.
Research into digital transformation underscores the severity of this problem. According to research presented in Manufacturing 2030 NAM report, and EY research about trends in discrete manufacturing and some others, while 68% of industrial company CEOs report increasing their investments in digital technologies, only 25% of manufacturers express confidence in their data’s organization and reliability. This gap highlights a fundamental issue: despite spending heavily on digital tools, many companies lack the foundational data technologies and approach to create a robust foundation for holistic data organization.
The lack of confidence in data is not just a technical issue but also a cultural and strategic one. When data is fragmented, inconsistent, or unreliable, it becomes difficult to build trust in the systems that rely on it. As a result, organizations may struggle to achieve the full potential of their digital investments.
The limitations of existing solutions
Traditional Product Lifecycle Management (PLM) systems have long been the backbone of data management in manufacturing. These systems were designed to serve as centralized repositories for product-related data, using relational database architecture to organize and store information. While they have been effective in managing structured data within individual companies, they fall short in addressing the complexities of modern manufacturing ecosystems.
One major limitation of traditional PLM systems is their reliance on tables and local identifiers. This rigid structure makes it difficult to adapt to changing requirements or integrate new data sources. The relational database model, while efficient for simple tasks, struggles to handle the dynamic and interconnected nature of modern manufacturing data.
Another issue is the single-tenant architecture of most PLM systems. These systems are designed for use within a single organization, making them poorly suited for collaboration across companies or supply chain networks. In an era where partnerships and external integrations are critical, this limitation becomes a significant barrier.
Traditional PLM systems also suffer from a lack of flexibility when it comes to data recombination. Once data is stored in a specific format, it is often difficult to repurpose or integrate it with other systems. This rigidity limits the ability of manufacturers to innovate or respond quickly to new opportunities.
Perhaps the most significant challenge is the disconnection between traditional PLM architectures and the broader vision of a “digital web.” While the future of manufacturing is increasingly defined by interconnected systems and seamless data flows, PLM systems remain rooted in siloed SQL databases and outdated architectures. This disconnect creates a gap between what manufacturers need and what traditional systems can deliver.
Elements of a future digital footprint
To address these challenges and build a more effective digital footprint, manufacturers must adopt new approaches to data management and system design. This transformation involves rethinking how data is structured, shared and used across the organization and beyond.
The role of graph models and linked data
One of the most promising innovations is the adoption of graph-based data models. Unlike traditional relational databases, graph models provide new ways to represent data models and data itself in a seamless, self-describing and robust way as nodes and connections. This approach is inherently more flexible, allowing data to be connected and recombined in ways that were previously impossible.
Graph models are particularly well-suited to the complexity of modern manufacturing data. They can represent relationships between components, processes and systems in a way that mirrors the real-world complexity of manufacturing operations. Most importantly this approach provides a mechanism to extend and modify the data as the scope and business requirements are growing. Graph models are extremely powerful for recombining data coming from multiple systems. Graph models make it easier to analyze data, identify patterns and derive insights that drive better decision-making.
Semantic web standards and knowledge graphs
Another critical element of the future digital footprint is the use of semantic web standards. Technologies like RDF (Resource Description Framework) and OWL (Web Ontology Language) provide a standardized way to describe and link data, making it more interoperable and machine-readable. These standards are the foundation of linked data, enabling seamless integration across systems and organizations.
At the center of this transformation is the knowledge graph, a universal information model that combines graph-based structures with semantic web standards. Knowledge graphs can represent complex relationships between data points, making them an ideal foundation for modern PLM architectures. They allow data from different sources to be connected seamlessly, creating a single source of truth that supports more intelligent decision-making.
Data transformation and process transformation
Data transformation is a key step in building a digital footprint. This involves moving from disconnected silos to a connected data ecosystem. Companies must consolidate data from multiple sources, link it to create a coherent picture and enable real-time collaboration. This shift from static documents to dynamic, interconnected data can unlock significant efficiency gains and foster innovation. It is important to highlight that doing so doesn’t mean the replacement of existing solutions. Modern graph-based data management systems are capable of capturing and containing data coming from multiple systems.
Process transformation goes hand in hand with data transformation. Traditional workflows often involve documents being passed around via email or through rigid approval processes. By shifting to data-driven workflows, companies can enable instant sharing, collaborative processes and real-time updates. This reduces inefficiencies, improves agility and allows manufacturers to respond more quickly to changing requirements.
Expanding the digital footprint for operational efficiency
Building a comprehensive digital footprint requires a holistic approach that combines strategic, technological and operational changes. It is not just about implementing new tools but also about rethinking how data is managed and used across the organization.
Strategy and cultural shift
The first step is a shift in mindset. Organizations need to move away from viewing information as static documents and start treating it as dynamic, interconnected data. This change requires not only new tools but also education and cultural change within the organization. Teams must understand the benefits of data-driven approaches and embrace a culture that values transparency, collaboration and innovation.
Technological foundations
From a technological perspective, creating an open and connected data architecture is essential. It is important to realize that the days of a “single database” serving a company are over. This includes adopting graph-based models, using semantic web standards and ensuring systems are designed to integrate seamlessly. The goal is to create a framework that can adapt to future needs and support continuous improvement.
Implementation and integration
Implementation is another critical area. Companies must focus on connecting existing data from legacy systems and operational sources, building new applications that enable more dynamic workflows and integrating these tools into existing processes. The aim is to create a seamless environment where data flows freely and supports smarter decision-making. It is important to emphasize that existing solutions represent a critical element in modern data architecture. By connecting (and not replacing them) and complementing them with new solutions, companies will be able to achieve much faster ROI and solve business problems faster.
AI and decision support
It would not be complete without mentioning AI and related data management tools. There are multiple places where AI tools can be used. But the most important thing is to think about how to feed AI tools with the most accurate data. AI tools can analyze data, identify patterns and provide insights that improve decision-making. However, these tools rely on a solid data foundation. Without reliable, connected data, AI systems cannot deliver their full potential.
The “data” is one of the most commonly used words in the lexicon of PLM minded people these days. What is important is to focus on the solid data management foundation, without that, all these new “data” solutions will be weak and not robust enough to support modern manufacturing environment and digital processes.
The way to transform the digital footprint through data transformation is essential for manufacturers looking to stay competitive in an increasingly complex and digital world. By addressing the limitations of traditional systems and adopting modern approaches like graph-based models, semantic web standards and knowledge graphs, companies can create a connected and efficient data ecosystem.
This transformation requires more than just new technology; it demands a shift in strategy, culture and processes. By embracing these changes, manufacturers can unlock new levels of operational efficiency, foster innovation and position themselves for long-term success in the digital era.